Predictive Analytics in Manufacturing: What It Means for the Future of Production
Written by Tom Czaban |
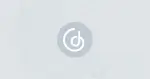
As production lines grow more complex and customer expectations rise, traditional manufacturing processes are reaching their limits. Today’s manufacturers must juggle competing demands — producing more with fewer resources, delivering top-quality products faster, and staying resilient amid supply chain disruptions and workforce challenges
The solution to these challenges? Data — and more specifically, predictive analytics. What once felt like guesswork is now a science, transforming uncertainty into opportunity. Using manufacturing data analytics software, teams can predict equipment failures, fine-tune production schedules, improve product quality, and build more agile supply chains.
In this article, we’ll explore predictive analytics' role in manufacturing, the technology behind it, and its real-world impact on production lines worldwide.
The Shift to Industry 4.0 and Smart Manufacturing
When people talk about the future of manufacturing, Industry 4.0 is often mentioned as a key driver of change. But what exactly is it?
Often referred to as the Fourth Industrial Revolution, Industry 4.0 is the next big leap in manufacturing, where advanced digital technologies create smarter, more connected production environments. It builds on previous industrial revolutions, which introduced mechanization, mass production, and automation, by integrating modern technologies like artificial intelligence (AI), machine learning (ML), and the Internet of Things (IoT) directly into manufacturing processes.
The impact of smart manufacturing is profound. According to McKinsey & Company, businesses embracing Industry 4.0 have reported:
- 30–50% reductions in machine downtime.
- 10–30% increases in throughput.
- 15–30% improvements in labor productivity.
- 85% more accurate demand forecasting.
The Role of Predictive Analytics in Smart Manufacturing
How does smart manufacturing create such transformational gains? Machines, sensors, and systems communicate with each other to gather vast amounts of data across the production floor, allowing manufacturers to monitor performance, identify inefficiencies, and make faster, more informed decisions. The result is a shift from traditional, reactive processes to proactive and predictive ones — and that’s where predictive analytics takes center stage.
Predictive analytics analyzes historical and real-time data to identify patterns and forecast future events. In traditional manufacturing, issues like equipment failures, quality defects, or supply chain disruptions tend to be ignored until after they cause problems. Predictive analytics flips this approach on its head. By constantly monitoring data streams from machines and production lines, it detects early warning signs of potential failures or inefficiencies, giving manufacturers the ability to act before disruptions occur.
Traditional vs. Smart Manufacturing
The table below offers a quick comparison of traditional manufacturing methods and smart processes like predictive analytics.
Aspect | Traditional Approach | Analytics Approach |
---|---|---|
Maintenance Strategy | Reactive or scheduled maintenance after failures occur. | Predictive maintenance anticipates failures and prevents downtime. |
Quality Control | Manual inspection or post-production checks. | AI-driven quality control detects defects in real time. |
Production Planning | Based on historical data and static schedules. | Dynamic adjustments based on real-time insights. |
Supply Chain Management | Responds to disruptions after they happen. | Proactively manages risks with AI-powered forecasting. |
Decision-Making | Relies on human intuition and experience. | Data-driven insights enhance decision-making. |
Cost Efficiency | Higher costs due to unexpected breakdowns and inefficiencies. | Reduced costs through optimized processes and minimized downtime. |
5 Key Benefits of Predictive Analytics in Manufacturing
Predictive analytics offers several key benefits that boost productivity, reduce costs, and enhance product quality. Let’s take a closer look at five of the most impactful advantages:
1. Predictive Maintenance: Reducing Downtime and Extending Equipment Life
Unplanned downtime is the enemy of efficiency. In traditional setups, manufacturers either perform maintenance on a fixed schedule or wait until a machine breaks down before taking action. Both approaches have major downsides: reactive maintenance leads to costly disruptions, while scheduled maintenance can be unnecessary and time-consuming.
Predictive maintenance solves this problem by continuously monitoring equipment through IoT sensors. These sensors track performance metrics, and predictive models analyze this to detect subtle changes that indicate potential failures. When a machine shows early signs of wear or malfunction, maintenance teams receive alerts, enabling them to fix the issue before it causes downtime.
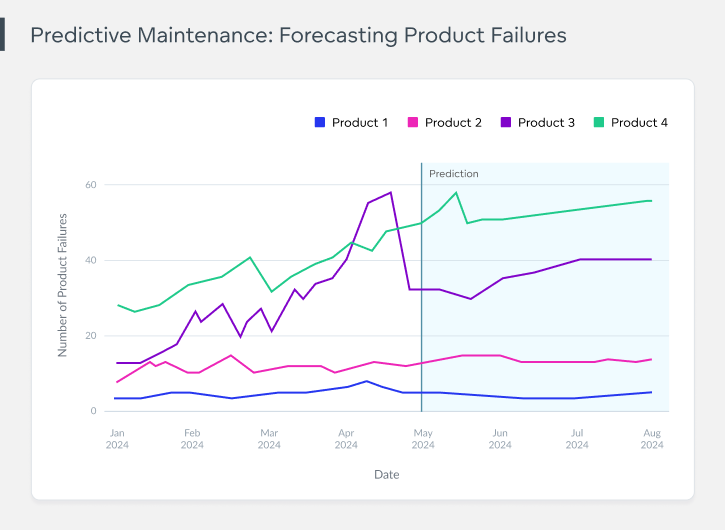
Predictive analytics example in manufacturing
2. Production Optimization with AI: Maximizing Efficiency
Manufacturing is a complex dance between machines, materials, and workers. When one part of the process slows down, it creates bottlenecks that ripple through the entire operation. Predictive analytics offers a smarter way to manage these complexities by analyzing production data to uncover inefficiencies and optimize workflows.
AI models forecast potential slowdowns by examining factors such as machine performance, staffing levels, and material availability. When a potential delay is detected, the system suggests adjustments, such as rerouting production or balancing workloads across different lines.
3. AI-Driven Quality Control: Catching Defects Before They Happen
In industries like aerospace, automotive, or pharmaceuticals, even minor defects can have massive consequences. Traditional quality control often relies on spot checks or end-of-line inspections, which can miss subtle defects and lead to product recalls.
Predictive analytics changes this by taking advantage of continuous, real-time quality monitoring. AI-powered models analyze production metrics to detect patterns that may indicate potential defects. With the help of AI tools, these can be turned into visualizations and dashboards. Operators can then receive alerts as soon as an anomaly is detected, allowing them to intervene before the defect affects the entire batch.
4. Supply Chain Resilience: Navigating Uncertainty
Supply chains are more complex and fragile than ever before. Global disruptions, from natural disasters to geopolitical tensions, can create delays and shortages that ripple through production lines. Predictive analytics helps manufacturers build more resilient supply chains by enhancing inventory management and improving demand forecasting.
By analyzing factors like financial market trends, supplier performance, and geopolitical risks, predictive models identify potential disruptions before they happen. Manufacturers can then adjust sourcing strategies, optimize inventory levels, or find alternative suppliers.
5. Energy Efficiency: Cutting Costs and Boosting Sustainability
Predictive analytics helps improve energy efficiency by uncovering usage patterns and optimizing energy loads in real time. For example, predictive models can recommend shifting energy-intensive processes to off-peak hours, when electricity rates are lower. They can also detect equipment that’s consuming more energy than usual, signaling the need for maintenance or upgrades.
Real-World Applications of Predictive Analytics in Manufacturing
Manufacturers often use dashboards to track key metrics like production output, equipment health, and quality control. These tools become even more powerful when combined with modern processes like predictive analytics, which is already driving real-world results across industries such as:
- Automotive
By using predictive maintenance tools, automotive manufacturers can track the condition of key components, such as engines or assembly line machines, and predict when they’re likely to fail. This allows manufacturers to schedule maintenance in advance, avoiding unexpected breakdowns that can halt production. As a result, unplanned downtime is reduced, machinery lifespan is extended, and production schedules are more reliable.
- Pharmaceuticals
Maintaining regulatory compliance and product consistency is critical in the pharmaceutical industry. With predictive analytics, pharmaceutical manufacturers can monitor environmental variables — such as temperature, humidity, and pressure — throughout the production process. By predicting potential shifts in these conditions that could lead to quality issues or non-compliance, manufacturers can take proactive action to correct them before they impact the product. This helps companies avoid costly compliance violations and recalls.
- Food and Beverage
Predictive analytics helps food and beverage manufacturers optimize production schedules and minimize waste, especially for perishable products. By analyzing data related to production cycles, inventory levels, and demand forecasts, predictive models can anticipate when products will be at risk of expiration or spoilage. This enables manufacturers to adjust production schedules and inventory management practices to meet actual demand, ensuring that products meet customer expectations.
The Technology Behind Predictive Analytics: AI, ML, and IoT
To fully appreciate the impact of predictive analytics, it’s essential to understand the underlying technologies that make it possible. As touched upon earlier, three key technologies — AI, ML, and IoT — are at the heart of this revolution. Let’s break these down further to better understand their role.
Machine Learning in Manufacturing
This is a subset of AI that enables systems to learn from data and improve over time without being explicitly programmed. In manufacturing, machine learning algorithms are continuously fed data from production lines, sensors, and equipment. These algorithms process vast amounts of information to identify patterns and trends that might otherwise go unnoticed. By learning from this data, machine learning systems can predict when machines are likely to fail, identify inefficiencies in workflows, and suggest improvements to production processes.
AI in Manufacturing
AI takes machine learning a step further by enabling real-time decision-making and the automation of complex processes. Modern BI tools powered by AI can process production data in real time, identifying inefficiencies and recommending immediate corrective actions. This capability is particularly valuable in environments where real-time adjustments are critical, such as high-speed production lines or industries with tight production deadlines.
AI also plays a significant role in optimizing workflows by dynamically adjusting production schedules, managing inventory, and even controlling robotic systems to handle tasks that require precision and consistency. With this in mind, is it any wonder that the AI manufacturing market is projected to reach $20.8 billion by 2028?
IoT in Manufacturing
IoT refers to the network of connected devices that communicate with each other and transmit data. In manufacturing, IoT devices are embedded in machinery, equipment, and production lines, collecting data on everything from machine performance to environmental conditions. This continuous flow of real-time data feeds directly into predictive analytics models, enabling manufacturers to monitor operations closely, detect anomalies, and take preemptive action.
Overcoming Implementation Challenges
Although predictive analytics offers significant benefits, implementing these advanced technologies comes with its challenges. Here are three of the most pressing:
1. Data Silos and Integration Issues
Legacy systems and older technologies often struggle to communicate with newer platforms, which means valuable data collected from various sources (e.g., machinery, sensors, inventory management systems) is stored in isolated systems. This fragmentation makes it difficult to obtain the holistic view of operations necessary for accurate predictive modeling.
To overcome this, manufacturers must develop effective integration strategies. This could involve investing in new, more flexible systems that can easily connect with existing infrastructure, or adopting middleware solutions that facilitate data flow between different platforms. A centralized data management strategy is also essential for breaking down silos: by ensuring data can flow freely across systems and departments, manufacturers can tap into the full potential of predictive analytics.
2. Skill Gaps
Implementing predictive analytics requires specialized knowledge, and many manufacturers face a shortage of skilled professionals who can build, manage, and refine predictive models. Data scientists and ML experts are crucial for developing algorithms that accurately predict outcomes and continuously improve over time.
Manufacturers can tackle this challenge by upskilling their workforce and partnering with external experts. Another effective solution is adopting a data analytics platform with AI features. This ought to provide user-friendly tools that don’t require deep technical expertise.
3. Change Management
Adopting predictive analytics requires more than just implementing new technologies: it requires a shift in mindset. Employees across all levels must be on board with the change. In many cases, resistance can arise due to fear of job displacement or a lack of understanding of how new technologies will fit into existing workflows. Addressing these concerns head-on and providing consistent support throughout the implementation process will increase the likelihood of a successful transition. Leadership should lead by example, encouraging a data-first approach and fostering an environment where data insights drive decision-making.
Future Trends: What’s Next for Predictive Analytics in Manufacturing?
Predictive analytics is evolving rapidly, bringing new capabilities that promise to revolutionize manufacturing even further. As technology advances, several key trends are emerging that manufacturers should keep an eye on:
- Predictive Maintenance 2.0: Enhanced accuracy in predicting equipment failures through deeper integration with IoT and real-time machine learning models.
- Hyper-Personalized Production: Predictive models that enable highly customized manufacturing processes based on shifting customer demands and market trends.
- Self-Optimizing Production Lines: Continuous feedback loops where predictive insights dynamically adjust machinery settings and workflows to maximize efficiency.
- End-to-End Supply Chain Forecasting: Predictive analytics will be leveraged to anticipate disruptions across the entire supply chain, ensuring resilience and just-in-time manufacturing.
How GoodData Can Help Meet Your Manufacturing Analytics Needs
GoodData’s comprehensive analytics solution is designed to meet the unique needs of manufacturers. Improve operational efficiency, minimize equipment downtime, and enhance product quality by making smarter, data-driven decisions at every stage of production. Request a demo to see how the platform can help you optimize your manufacturing processes and stay ahead of the rest.
Frequently Asked Questions (FAQs)
What is predictive analytics in manufacturing? Predictive analytics in manufacturing uses historical and real-time data to forecast future outcomes, helping businesses anticipate issues like equipment failure or production delays before they happen.
What is an example of predictive analytics in manufacturing? An example is predictive maintenance, where sensors monitor equipment to predict failures, allowing manufacturers to fix issues before they cause unplanned downtime.
How is data analytics used in manufacturing? Data analytics is used to optimize processes, improve quality, predict maintenance needs, enhance supply chain management, and boost overall productivity.
How is AI used in manufacturing? AI is used to analyze vast amounts of data, automate processes, optimize production schedules, and improve decision-making, leading to faster, more efficient manufacturing operations.
What are the problems with AI in manufacturing? Challenges with AI in manufacturing include data integration issues, high upfront costs, lack of skilled workers, and the need for ongoing maintenance of AI systems.
Written by Tom Czaban |