4 Industry Examples of Leveraging Predictive Analytics
Written by Sandra Suszterova |
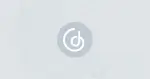
Predictive analytics helps businesses anticipate trends, seize opportunities, and prevent losses. With increasing data and user-friendly software, this technology is becoming essential for strategic planning and boosting profitability.
What is predictive analytics?
Predictive analytics is a method that uses data to predict future trends. It encompasses a variety of statistical techniques, AI, and machine learning models, which analyze both current and historical data to forecast future trends and behaviors. This approach is crucial for decision-making across various industries as it helps companies and organizations predict outcomes and effectively prepare for future developments.
How does predictive analytics work?
Predictive analytics transforms raw data into actionable insights, guiding business decision-making processes. Here's how you can effectively implement this approach:
- Define goals: Start by clearly identifying the outcomes or trends you want to predict, for example, sales volumes, customer behavior, or risk factors. This clarity will shape the predictive models for more relevant insights.
- Integrate data: Collect data from internal sources (e.g., sales records) and external sources (e.g., market trends). Organize this into a unified dataset to complete the predictive model's foundation.
- Set up analytics tools: Link your datasets to an analytics tool to create metrics, dashboards, and reports. This setup aids data visualization and prepares it for deeper analysis, allowing continuous monitoring and interaction with data trends.
- Build and train models: Either build and train custom predictive models or utilize pre-built models provided by AI analytics tools (e.g., GoodData).
After making decisions based on predictions, the cycle begins anew: you collect new data, monitor the results, tweak your models, and once again make a decision. This cycle continuously enhances prediction accuracy and decision effectiveness.
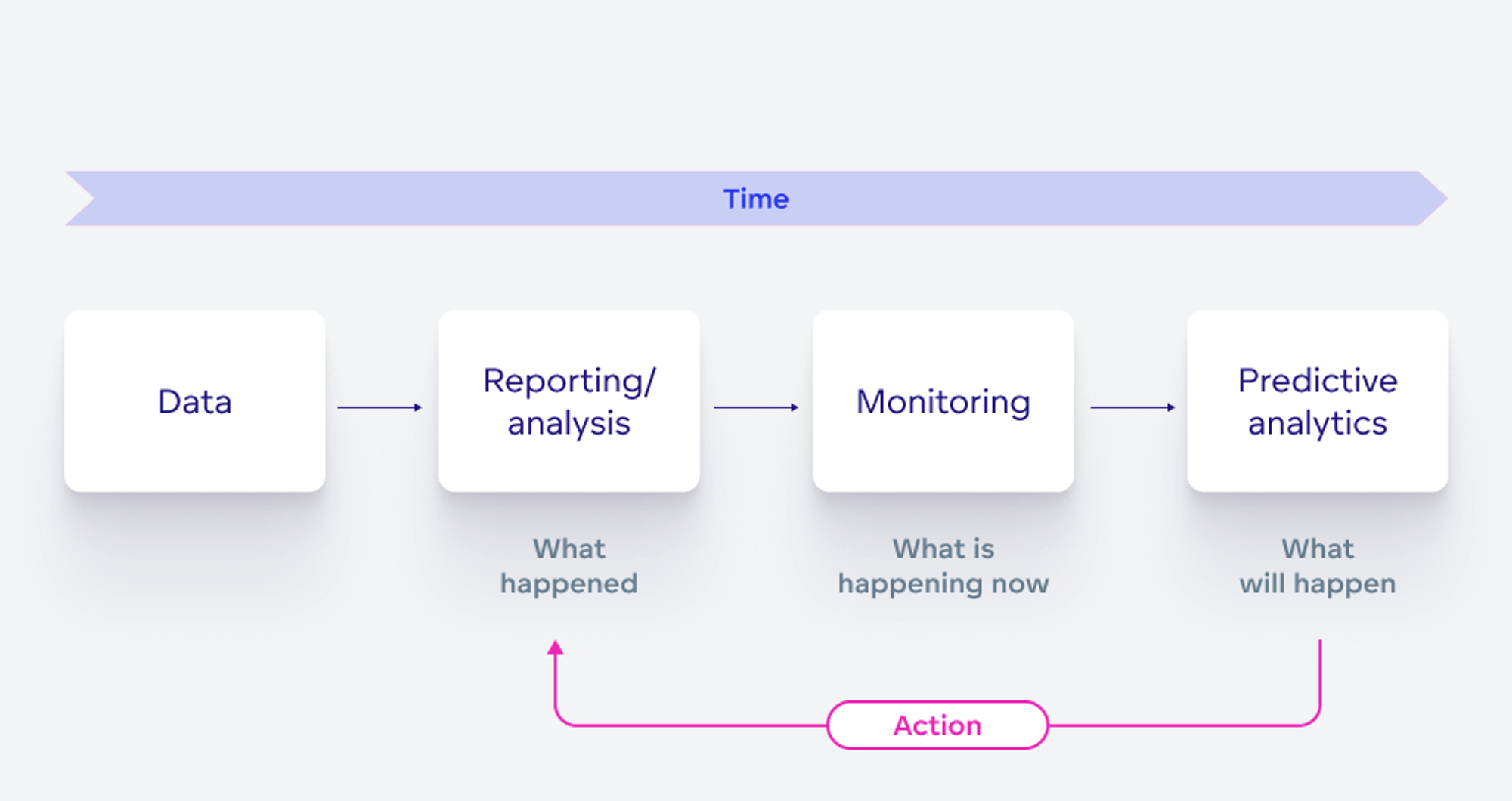
Predictive analytics position
Predictive analytics techniques
Predictive models come in two main types: classification and regression. Classification models sort data into categories, such as approving or denying loans, while regression models predict continuous values like future sales. Additionally, neural networks (a type of machine learning) model complex relationships, such as diagnosing diseases from medical images.
Various analytics tools now incorporate AI and machine learning (ML) patterns into their solutions, enabling self-service data science with one-click access to predictive analytics techniques. These predictive capabilities might include:
- Forecasting provides informed estimates about future events based on historical data. You can see predicted values for specific time frames in your visualizations by defining future periods. You can directly access the predictive models in Jupyter Notebook for more advanced forecasting using the analytics software – as demonstrated in this video.
- Data clusters group a set of objects so that objects in the same group (or cluster) are more similar to each other than those in different groups. Additionally, you can access, modify, test, and deploy your clustering model directly from a Jupyter Notebook – as demonstrated in this short snippet.
- By detecting outliers you can identify data points that significantly differ from the rest of the data. This helps to isolate unusual patterns or errors within the dataset.
In addition to these predictive capabilities, you can integrate the solution with existing ML services, such as Amazon SageMaker. This integration enables you to build, train, and deploy ML models within your visualizations and dashboards. For a practical example, check out How To Forecast Gross Profit with GoodData and AWS SageMaker.
Predictive analytics examples
Let's explore how predictive analytics is revolutionizing industries, using key examples that showcase its impact on strategic decision-making and efficiency. This technology is widely applicable across different industries, significantly enhancing strategic planning and decision-making processes.
Predictive analytics in healthcare
Predictive analytics in healthcare uses current and historical data to help healthcare professionals make informed decisions, forecast health trends, and manage the spread of disease – ultimately improving patient care and saving lives.
The predictive analysis relies on data from medical records, health surveys, and connected devices, processed through healthcare analytics software. Stakeholders such as hospitals, doctors, and pharmaceutical companies can then use these insights to improve health outcomes.
Use cases of predictive analytics in healthcare
There are many healthcare fields where predictive analytics can be applied. Below are a few examples of predictive analytics use cases in healthcare:
- Clinical research: Testing antibiotics and developing rapid vaccines for COVID-19 and seasonal flu; studying connections between diet, heart disease, and smoking.
- New treatment development: Analyzing effectiveness and patient outcomes to innovate treatments.
- Disease prevention: Forecasting and mitigating epidemics; identifying disease predisposition to act ahead of time.
- Improving the treatment success rate of surgeries and medications: Particularly in chronic conditions such as cancer.
- Healthcare device maintenance: Predictive analytics facilitate proactive medical device maintenance, prevent failures before they occur, and ensure continuous operational efficiency.
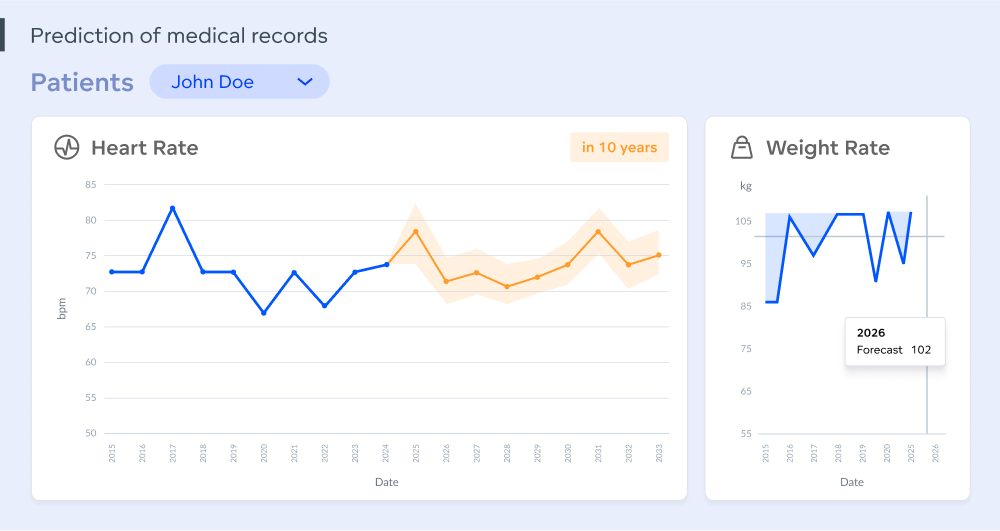
Predictive analytics example in healthcare
There are several benefits of predictive analytics in healthcare, including improving patient outcomes by analyzing data and medical histories for accurate diagnoses and improved treatment plans. Predictive algorithms can aid with clinical decision-making, identify at-risk patients, and help to maintain essential services. They are also crucial in detecting and preventing healthcare fraud such as prescription and billing – as they identify unusual patterns early to safeguard resources.
Predictive analytics in insurance
Predictive analytics in insurance applies statistical algorithms and machine learning techniques to forecast future events and behaviors in the insurance industry. It helps insurers assess risks and make informed decisions in underwriting, pricing, claims handling, and fraud detection.
Insurance sector data can include personal customer information, policy details, claims data, telematics from vehicles, smart devices, and more. This data is relevant for underwriters, claims adjusters, fraud investigators, technology providers, and policyholders.
Use cases of predictive analytics in insurance
Predictive analytics examples in insurance include:
- Risk and fraud prevention: Detecting fraudulent claims early by analyzing patterns and anomalies in the data.
- Increased customer engagement: Using predictive analytics to provide personalized services and understand customer preferences and behaviors.
- Forecasting customer risk: Employing predictive models to identify regions or demographics that may present higher future risks.
- Policy optimization: Using historical data and algorithms to tailor policies to individual risk profiles.
- Claim management: Detecting outliers that significantly deviate from expected patterns, aiding in efficient claims processing and fraud detection.
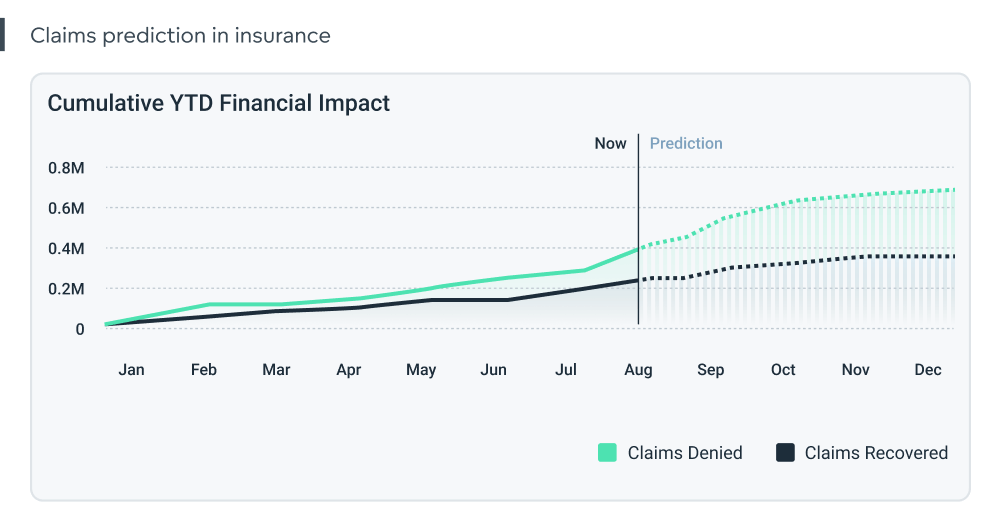
Example of predictive analytics in insurance
The above use cases offer insurance companies significant benefits. Predictive analytics improves operations by reducing costs and enhancing efficiency, while AI-powered analytics tools allow insurers to swiftly detect fraud, extract detailed insights from data, and gain a deeper understanding of customer behaviors and risk profiles for more personalized services.
Predictive analytics in manufacturing
Predictive analytics in manufacturing uses current and historical data to forecast future outcomes through statistical models or advanced predictive modeling, ensuring smooth production and high standards within the manufacturing industry.
Manufacturing data comes from sensors, IoT devices, production lines, supply chains, and maintenance records. It is crucial for production managers, maintenance teams, quality control staff, and supply chain managers to predict and optimize future outcomes.
Use cases of predictive analytics in manufacturing
Using predictive analytics in manufacturing enables:
- Predictive maintenance: Anticipate equipment failures and schedule repairs only as needed to avoid unexpected downtime.
- Quality assurance: Real-time analysis of production data highlights deviations from standards, allowing for quick fixes and less waste.
- Supply chain optimization: Predict delays and adjust sourcing and logistics ahead of time to maintain smooth operations.
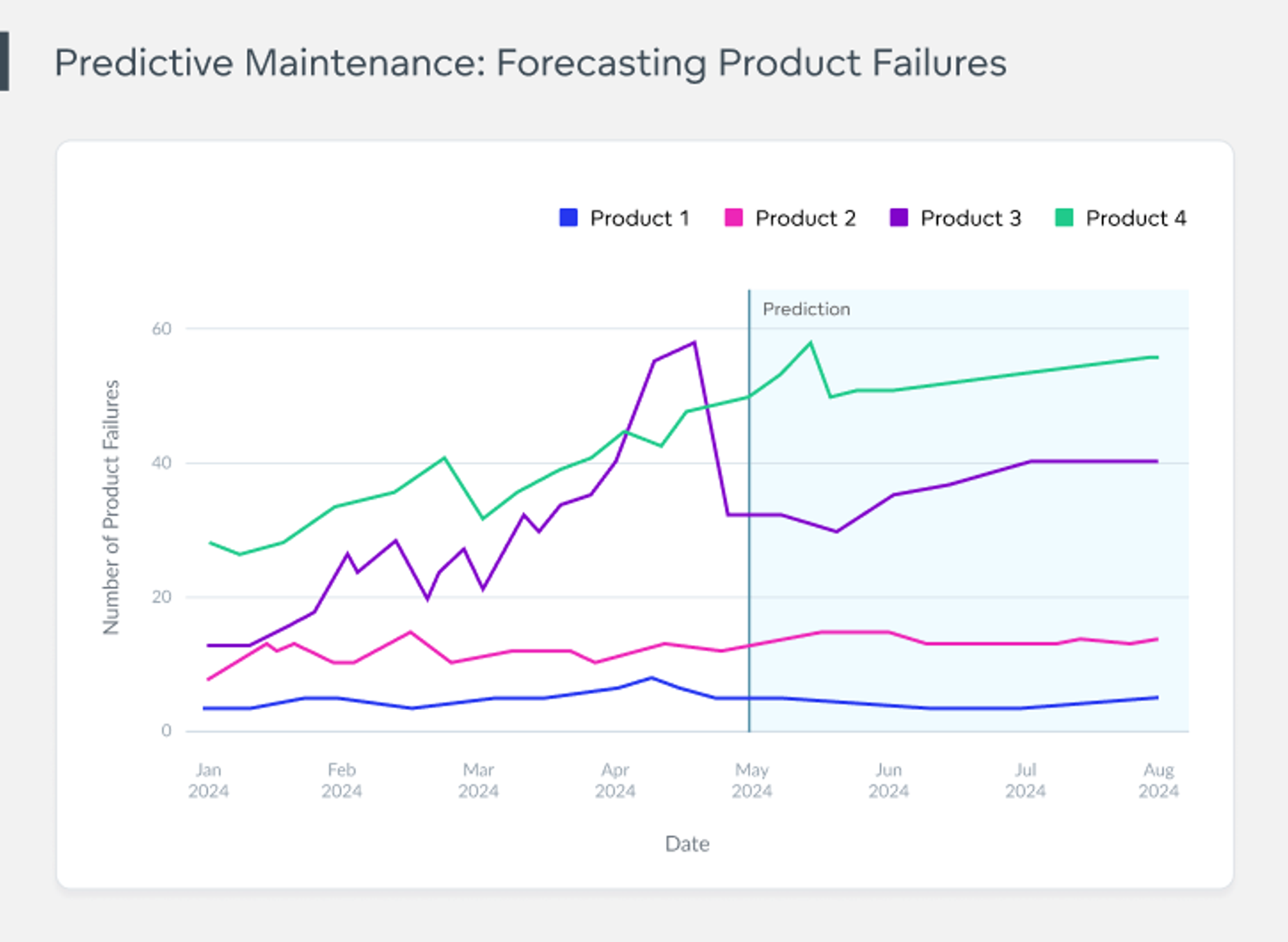
Predictive analytics example in manufacturing
These use cases primarily benefit cost reduction: minimizing downtime, improving production quality, and optimizing logistics lead to significant cost savings across production processes.
Predictive analytics in marketing
Predictive analytics in marketing uses statistical techniques, AI, and machine learning to analyze marketing data. It helps marketers understand customer behavior, predict trends, and make data-driven decisions.
Predictive marketing analytics leverages customer demographics, transaction histories, social media activity, browsing data, and reviews, to help marketing, sales, and customer service teams refine strategies, boost engagement, and optimize campaigns for greater efficiency.
Use cases of predictive analytics in marketing
Predictive marketing analytics has various use cases, where the key purpose is to optimize marketing efforts:
- Predict consumer trends: Analyzing patterns in large datasets enables businesses to anticipate market trends and adjust their offerings accordingly.
- Customer segmentation: Clustering can be used to differentiate customers into distinct groups based on similar characteristics, allowing for more targeted marketing approaches.
- Customized campaigns: Personalized messages and offers can be created, based on predicted customer preferences and behaviors.
- Customer churn reduction: This can be achieved by using predictive analytics to identify at-risk customers and implementing strategies to retain them.
- Lead prioritization: Scoring and ranking leads based on the predicted likelihood of conversion enables sales teams to concentrate on the most promising prospects.
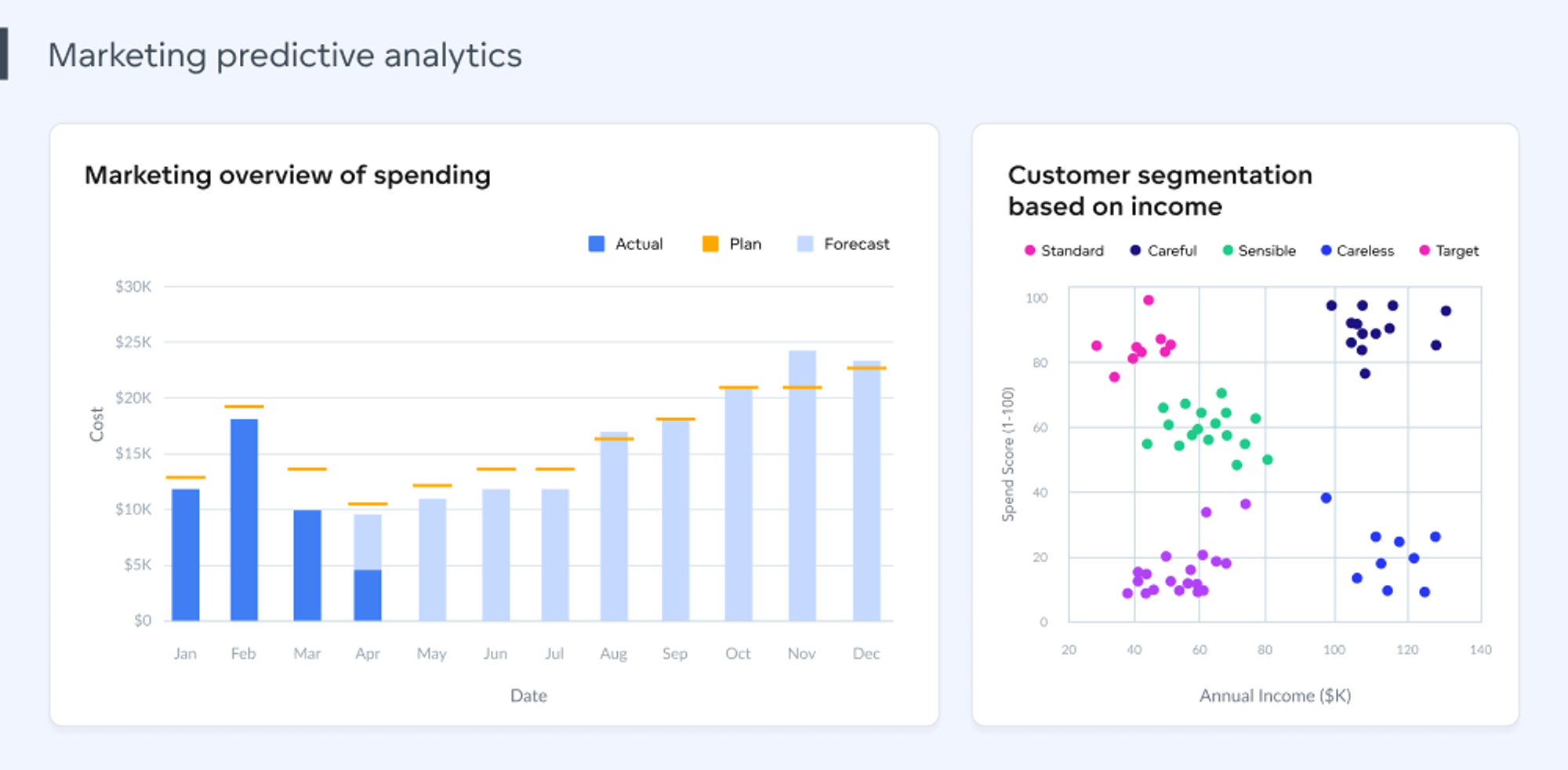
Marketing predictive analytics example
Such use cases of predictive analytics in marketing lead to significant benefits:
- They enable businesses to allocate resources more efficiently by focusing on high-ROI strategies.
- Enhance the customer experience through personalized recommendations tailored to individual preferences.
- Help identify new prospects similar to the company's best existing customers.
Harness predictive analytics with GoodData
Designed to optimize business processes and enhance decision-making, GoodData’s cloud-based analytics platform offers advanced data exploration and predictive analytics capabilities. It enables users to generate actionable insights and forecast trends. To try these features yourself, sign up for a free 30-day trial.
Alternatively, if you have questions or want to see real-time use cases, request a demo for a platform walkthrough.
Written by Sandra Suszterova |