How can we trust the numbers produced by AI systems?
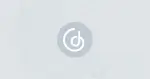
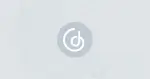
Generative AI-powered tools are appearing more and more outside the initial experimental zone and finding their way to real business settings. They are evolving from "toys" and "gadgets" into the category of essential "tools". Tools need to be precise and reliable. A carpenter needs to have a reliable hammer or accurate saw. He shouldn’t question the hammer's ability to drive the nails into the wood. Similarly, in the business world, there's no time and place to doubt whether the calculator gave you the correct number. Businesses rely on correct numbers. The trustworthiness of these numbers is not just a convenience; it's a necessity.
Trust can be a tricky challenge with all this AI development going on. It's typically not a big deal when Midjourney creates an unexpected image or when ChatGPT misunderstands the prompt and suggests irrelevant ideas. With such creative tasks, users ask the generative AI tool to try again or adjust the prompt. The problem becomes very real when the business user asks the AI-powered tool to present some hard numbers - e.g., the number of products their company sold over the past year or the revenue composition across product categories. The result is about something other than being likable or not - it's about being true. Incorrect numbers presented with AI's infinite confidence to unaware users could result in significant business damage.
How can we create AI-powered tools that convey trust? Generative AI tools are built on large language models (LLMs) - these are black boxes from their very definition. They aren’t transparent, and from any point of view, that is not a good start for building trust.
We need to be as transparent as possible about how the AI tool came to its conclusion – about their “thought process”. Convey the message that the AI tool is crunching your numbers, not making them up.
Be honest about the AI’s capabilities
Start with being honest and clear about what an AI system can do. Be sincere about its capabilities. Set the expectations about what the AI system is designed for. The field of AI is currently very dynamic and generates a ton of false information, misconceptions, or unreasonable fears. Therefore, users might come to your tool with prejudices or unrealistic expectations. Manage the expectations from the start so the users are not caught off guard. Clearly specify the focus of the AI system and what kind of output the user can expect.
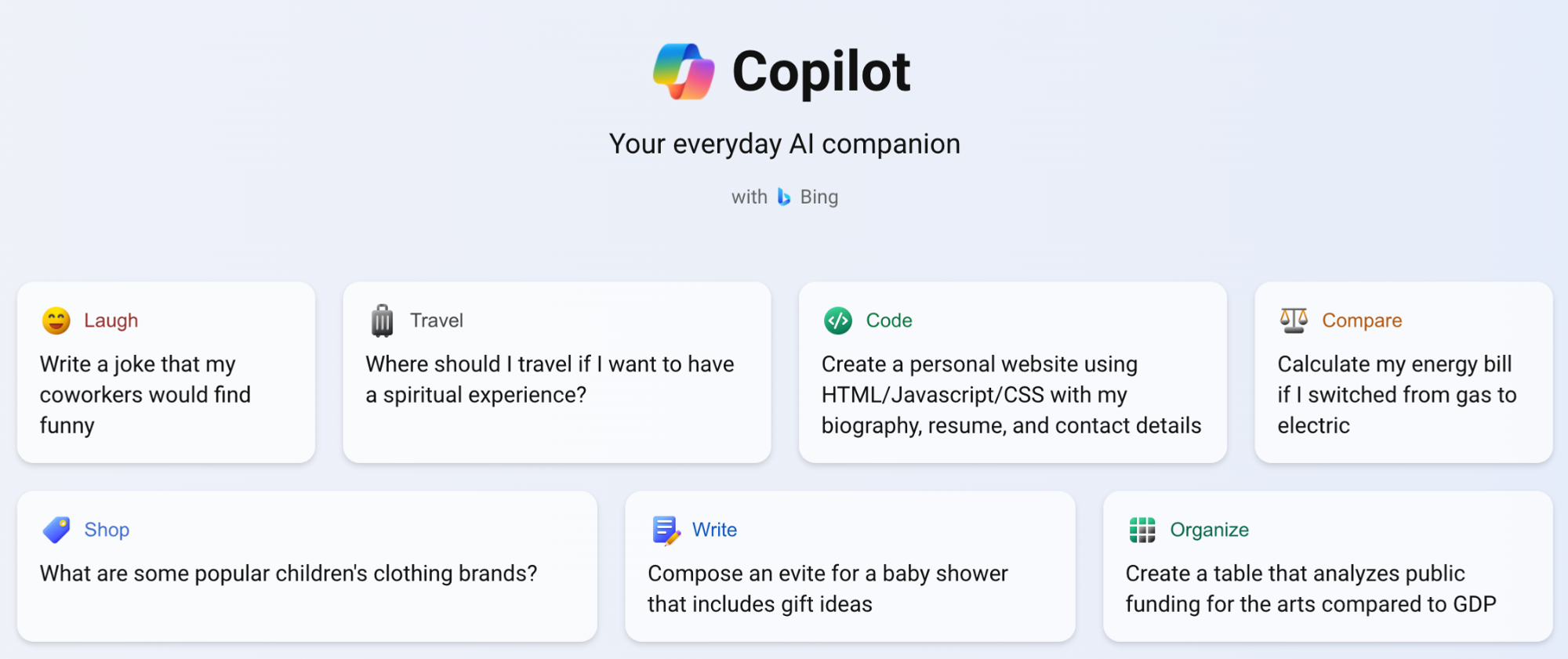
Set the right expectations
Next, make clear how well the system can do what it can do. Users might have their expectations set from different tools, so they may not realize how often the AI system makes mistakes, even in the tasks it is designed for. Current generative AI systems often hallucinate and confidently claim half-truths or utterly false information as correct so it is important to remind users to check the results. AI models also like to lie and fabricate backstories to get approval from their users.

Make it clear that AI is crunching real numbers
The source of data is not as important (and downright impossible to tell) when generating a creative output like a joke, poem, or image. However, it becomes very important once the user starts to use the gen AI tool to search for real-world information.
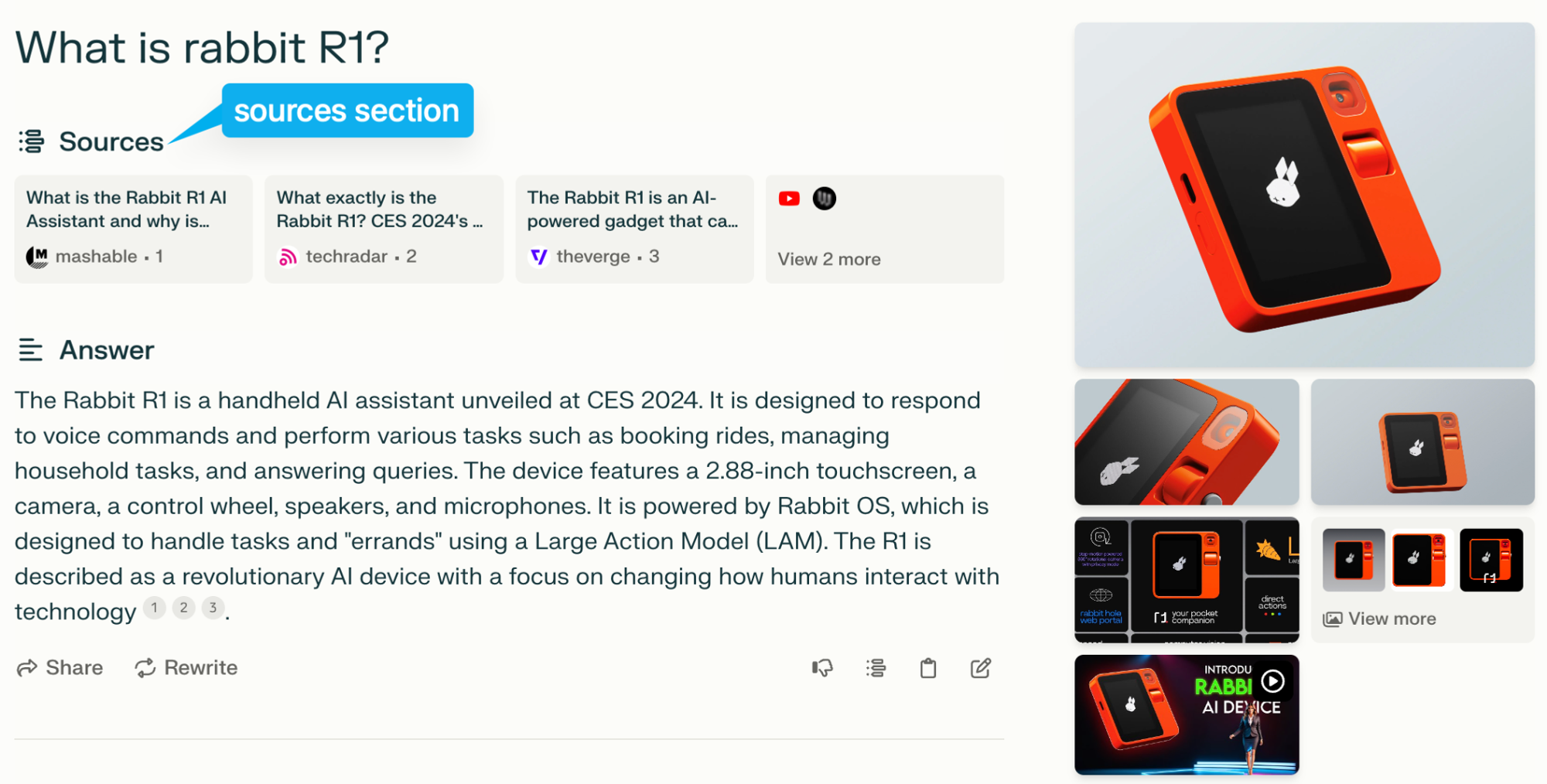
When we move one step further into the realm of accurate business data, transparency about the data sources is the main building block of trust. With business use cases, it needs to be crystal clear that the AI tools are not making the information and numbers up, but they are crunching your company's real data and calculating the results. If you want users' trust, you need to explain each step and show how the AI tool got to the result. Again, it's about showing that it's not making them up. This layer of transparency allows users to actually trust the result by quickly checking where the numbers are coming from.
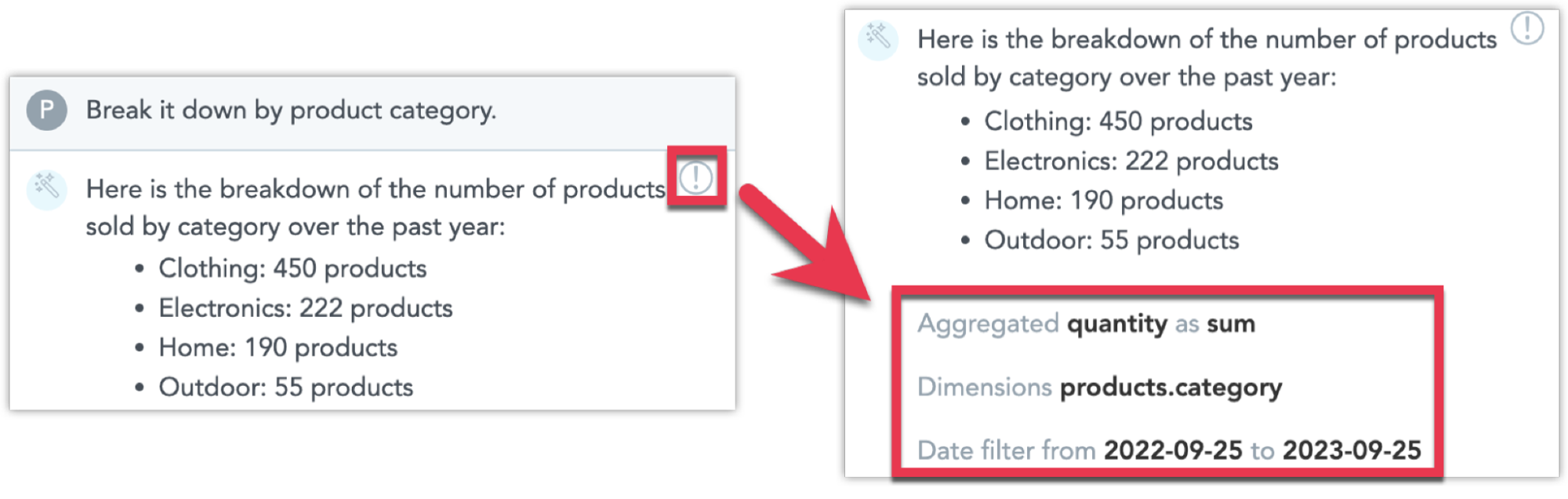
Ensure data privacy in business AI
When talking about business use cases, it's impossible not to mention the problem of data security and privacy. Companies building generative AI tools are not known for being particularly subtle about getting the training data for their models, so, understandably, business users are very cautious about their companies' data. One doesn’t need to search too hard to find one or two examples of such behavior. There, it must be clear that the AI tool is touching the company data to retrieve the desired results, and those data are not used for anything else – especially not for training the AI models.
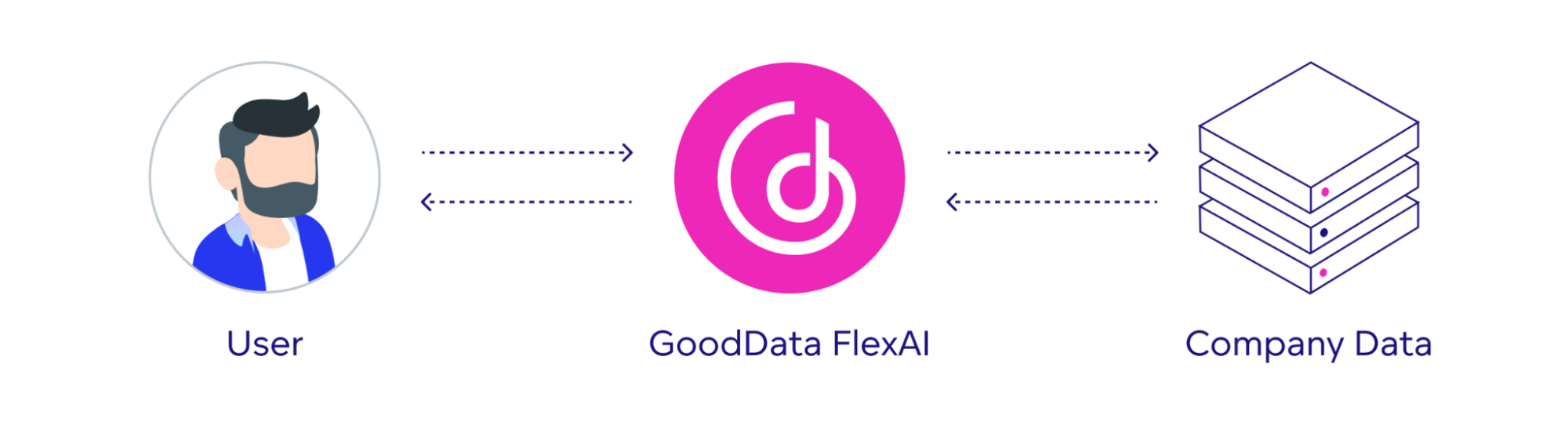
Building users' trust in the AI tool is challenging and very easy to break in the same way, as breaking trust between business partners, co-workers, or friends. A bad reputation is hard to fix, if not impossible. So, always think twice about the choices that might betray users' trust in your AI-powered product. Trust is simply the essence of business AI tools, and without it, generative AI will stay in the realm of toys and creative companions.
Learn More
Together with my colleagues at GoodData, we are working hard to deliver AI to the hands of business intelligence users. If you would like to learn more, here’s a simple recipe for a serverless AI assistant. Are you interested in trying the latest business AI-powered data analytics tool from GoodData on your own? You can - just sign up for the GoodData Labs here.