AI Security in Data Analytics: Safeguarding Data Integrity and Ensuring Compliance
Written by Natalia Nanistova |
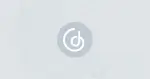
As artificial intelligence (AI) reshapes the landscape of data analytics, businesses are presented with unprecedented opportunities to extract valuable insights from their data. AI tools like intelligent search, natural language processing (NLP), and predictive analytics enable organizations to make smarter, faster decisions, automate processes, and drive innovation. However, this technological leap forward also comes with significant responsibilities, particularly concerning AI security.
AI security is not merely about protecting data from external threats. It involves safeguarding the entire ecosystem — ensuring that AI models are secure, accurate, transparent, and compliant with regulatory standards. As businesses become more reliant on AI to power critical decisions, failing to address these concerns could lead to reputational damage, legal consequences, and loss of stakeholder trust.
In this article, we examine the essential aspects of AI security in data analytics, outline best practices that businesses should adopt, and explore how GoodData’s platform ensures security, compliance, and transparency across its AI-powered services.
The Rise of AI in Data Analytics: Opportunities and Challenges
AI is fundamentally changing how businesses use data, enabling organizations to extract and deliver insights in ways that were previously unimaginable. AI’s ability to process vast datasets in real-time allows businesses to make data-driven decisions with greater speed and accuracy. While AI’s potential is vast, its integration into analytics systems also brings unique challenges.
The Growing Complexity of AI Models
One of the first hurdles businesses face with AI-powered analytics is the complexity of the models themselves. Many AI systems, especially machine learning models, operate as "black boxes." These models may produce accurate outputs, but the underlying processes that drive those outputs are often opaque. Without clear visibility into how AI models make decisions, businesses risk unintentionally overlooking errors, bias, or misinterpretations that could have significant real-world consequences.
For AI to be trustworthy and effective, transparency is crucial. Organizations must ensure that AI’s decision-making processes are explainable, accountable, and auditable to build stakeholder trust and comply with emerging regulatory requirements.
Ethical Considerations: Mitigating Bias and Ensuring Fairness
As AI systems learn from vast amounts of data, there is a real risk of perpetuating biases inherent in the data. AI models can unintentionally reinforce existing societal biases if they are trained on flawed or biased datasets. In sectors such as finance, healthcare, and human resources, biased AI outputs can lead to unethical decisions, damaging individuals and businesses alike.
To avoid this, businesses must be proactive in addressing bias in AI models. This includes using diverse, representative data, regularly auditing AI systems for fairness, and ensuring that model outputs are continually validated to meet ethical standards.
Navigating Regulatory and Compliance Challenges
As AI becomes more pervasive, the regulatory landscape continues to evolve. Data privacy laws such as GDPR, CCPA, and others are tightening the rules for data handling, especially when personal data is involved. AI systems often require large volumes of data, including sensitive information, and businesses must ensure their systems comply with these stringent regulations. Failing to comply can result in costly fines, legal disputes, and lasting reputational damage.
Beyond compliance, organizations must also stay ahead of emerging regulations specifically targeted at AI technologies. These regulations focus on ensuring AI systems are used responsibly, ethically, and transparently. Businesses must implement strong governance frameworks to ensure their AI systems meet current and future compliance standards.
Scalability and Integration with Existing Systems
As AI continues to scale, integrating AI models with existing data infrastructure presents significant challenges. Businesses must not only ensure that their systems can handle large volumes of data but also maintain security and privacy standards as they scale. For many organizations, this means revisiting data governance models, ensuring secure access to sensitive data, and maintaining the integrity of data across multiple platforms.
Effective integration requires a deep understanding of the technological architecture, ensuring that AI systems are aligned with the business’s broader data infrastructure. This will allow businesses to unlock the full potential of AI without compromising on security or operational efficiency.
Why not try our 30-day free trial?
Fully managed, API-first analytics platform. Get instant access — no installation or credit card required.
Get startedAI Security Best Practices: Building a Secure Framework
To harness AI’s potential while managing its risks, businesses must adopt a comprehensive approach to AI security. Below are some critical best practices that organizations should consider when building secure AI frameworks.
#1 Data Privacy and Governance
Data privacy is paramount when working with AI. Given that AI systems rely heavily on large datasets, organizations must implement strict measures to protect sensitive data. Data should be anonymized and encrypted to protect it from breaches or unauthorized access. Additionally, businesses must ensure their data governance practices are robust, defining clear rules about data access and usage, and adhering to privacy regulations.
#2 Explainability and Transparency
For businesses to confidently adopt AI, the technology must be explainable. Users should be able to trace how AI models arrive at their conclusions, enabling organizations to audit outputs for accuracy and fairness. By prioritizing transparency, businesses can reduce the "black box" effect and gain deeper insights into their AI models' behavior, enhancing trust and accountability.
#3 Bias Mitigation
Addressing bias is an ongoing process. AI models should be regularly assessed for potential biases and adjusted to mitigate them. This involves retraining models on more diverse datasets, implementing fairness criteria, and testing AI systems to ensure they provide equal treatment across all demographic groups.
#4 Access Control and Real-Time Monitoring
AI systems should include granular access control features to restrict sensitive data access to authorized users only. Real-time monitoring is also crucial, allowing businesses to detect and respond to any anomalies or unauthorized activity as it happens. This ensures that data and insights remain secure and compliant.
How GoodData Ensures AI Security in Data Analytics
At GoodData, we take AI security seriously, recognizing that businesses need reliable, secure, and transparent analytics platforms to leverage AI without compromising security. Here’s how we ensure our AI-powered platform stays secure and compliant.
Granular Access Controls and Real-Time Monitoring
GoodData offers fine-grained access controls to ensure that only authorized users can access sensitive data. This, combined with real-time monitoring capabilities, helps detect any suspicious activity, ensuring that your data remains protected at all times.
The Semantic Layer: Reducing AI Hallucinations
One of the unique advantages of GoodData’s platform is its semantic layer, which helps reduce AI “hallucinations” — incorrect or nonsensical AI outputs. By structuring data definitions and business rules, the semantic layer ensures that AI-generated insights are based on accurate, well-understood data, greatly reducing the risk of erroneous conclusions.
No Direct Submission of Raw Data to OpenAI
While GoodData leverages OpenAI's GPT-3.5 for features like Smart Search and AI Assistant, we take great care to ensure that no raw company data is submitted to OpenAI. Only metadata is sent to the LLM, keeping your data secure within your environment and minimizing exposure to external risks.
Auditability and Transparency in AI Interactions
GoodData allows users to audit all AI interactions, providing full visibility into the prompts and responses generated by AI models. This transparency ensures that users can trace how AI-driven decisions are made, enhancing accountability and trust.
See GoodData AI in Action
Ready to discover how GoodData's AI-powered platform can transform your data analytics?
Take the tour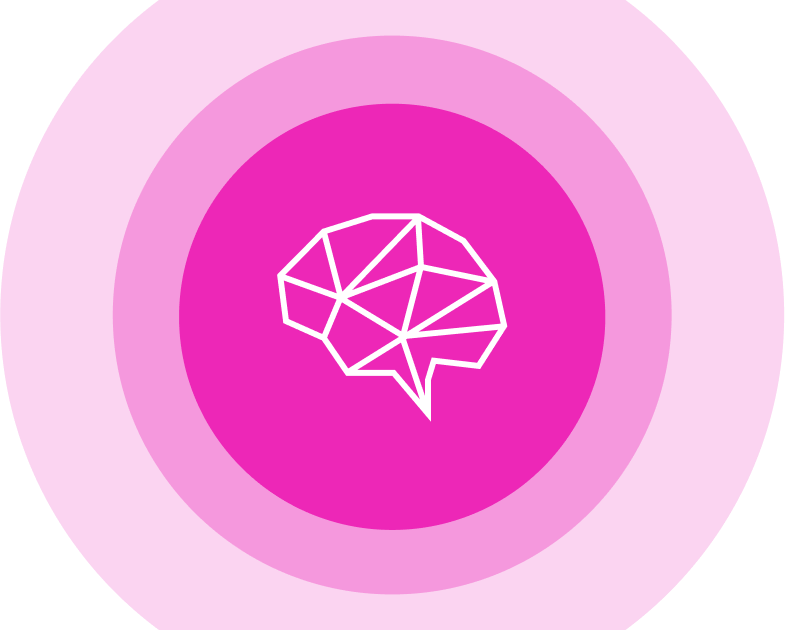
Conclusion: The Future of AI Security
As AI continues to evolve, ensuring robust security, privacy, and compliance will remain crucial for organizations looking to harness its power. With GoodData’s comprehensive AI security features, businesses can confidently leverage AI to drive innovation while safeguarding data, ensuring compliance, and maintaining transparency.
The future of AI in data analytics is bright, but only if organizations approach it with a clear commitment to responsible and secure practices. By implementing effective security measures and ethical guidelines, businesses can unlock AI’s full potential without compromising trust, compliance, or security.
Written by Natalia Nanistova |