5 Common Analytics Challenges for Snowflake Users
Written by Harry Dix |
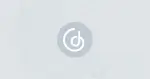
There are a plethora of tools and platforms to choose from when it comes to building dashboards with Snowflake data. For building interactive analytics apps with Snowflake, there is GoodData.
GoodData and Snowflake make an excellent combination for running your analytics app. Your next question is why, right? The answer is a bit long-winded but read on to learn about the five unique use cases GoodData provides to support Snowflake data users.
1. Eradicate Change-request Overload
The Situation
In analytics, one size doesn’t fit all. End users will always be looking for something directly suited to their needs (i.e., a different view of the data). This leads to your team will quickly become inundated with customization requests.
GoodData Solution
This is where multi-tenant architecture, a well-known GoodData staple, becomes a necessity. By providing separate workspaces — dedicated spaces where users can analyze their data and view their dashboards — for each client company or user group, you can easily enable end-user customizations of dashboards and reports while ensuring that each group’s data is separate and secure. On top of this, with plans priced per workspace rather than per user and the flexibility to add unlimited users per workspace, you can quickly and easily scale your product along with your Snowflake data warehouse.
2. Scale Analytics Alongside Snowflake Data Storage Without Sacrificing Performance
The Situation
Whether you plan to roll out analytics internally to employees or externally to customers, one of the main goals for your analytics solution will likely be to provide analytics to as many of your end users as possible. However, the flipside to this is that as your end-user uptake increases, so do the performance requirements of your data storage and your analytics. In addition, successful analytics applications are quite taxing from an operational perspective. As your application gains traction, you will soon see data volumes and concurrent user numbers grow, along with the prevalence of peak usage times.
GoodData Solution
In this instance, elastically scalable analytics is needed to complement your Snowflake data warehouse. GoodData’s elastic scalability efficiently scales by data volume, user number, and cost; so as your Snowflake data storage grows, your analytics and user numbers can scale along with it — without sacrificing performance.
Want to see what GoodData can do for you?
Get a guided tour and ask us about GoodData’s features, implementation, and pricing.
Request a demo3. Leverage Reusable Metrics to Empower End Users
The Situation
While multi-tenant architecture is one primary requirement for providing self-service analytics, another challenge is understanding who your end users will be. They likely won’t all be analysts by profession, which is why every step towards ease of customization is valuable. It further helps to prevent customization requests that would otherwise go to your product, support, or professional services teams.
GoodData Solution
GoodData’s solution is to implement reusable metrics. Reusable metrics is the most effective way to achieve ease of customization. By creating a semantic model and defining base metrics that your end users can later use when creating their specific metrics as simple arithmetic expressions, your end users can manage their analytics efficiently and confidently.
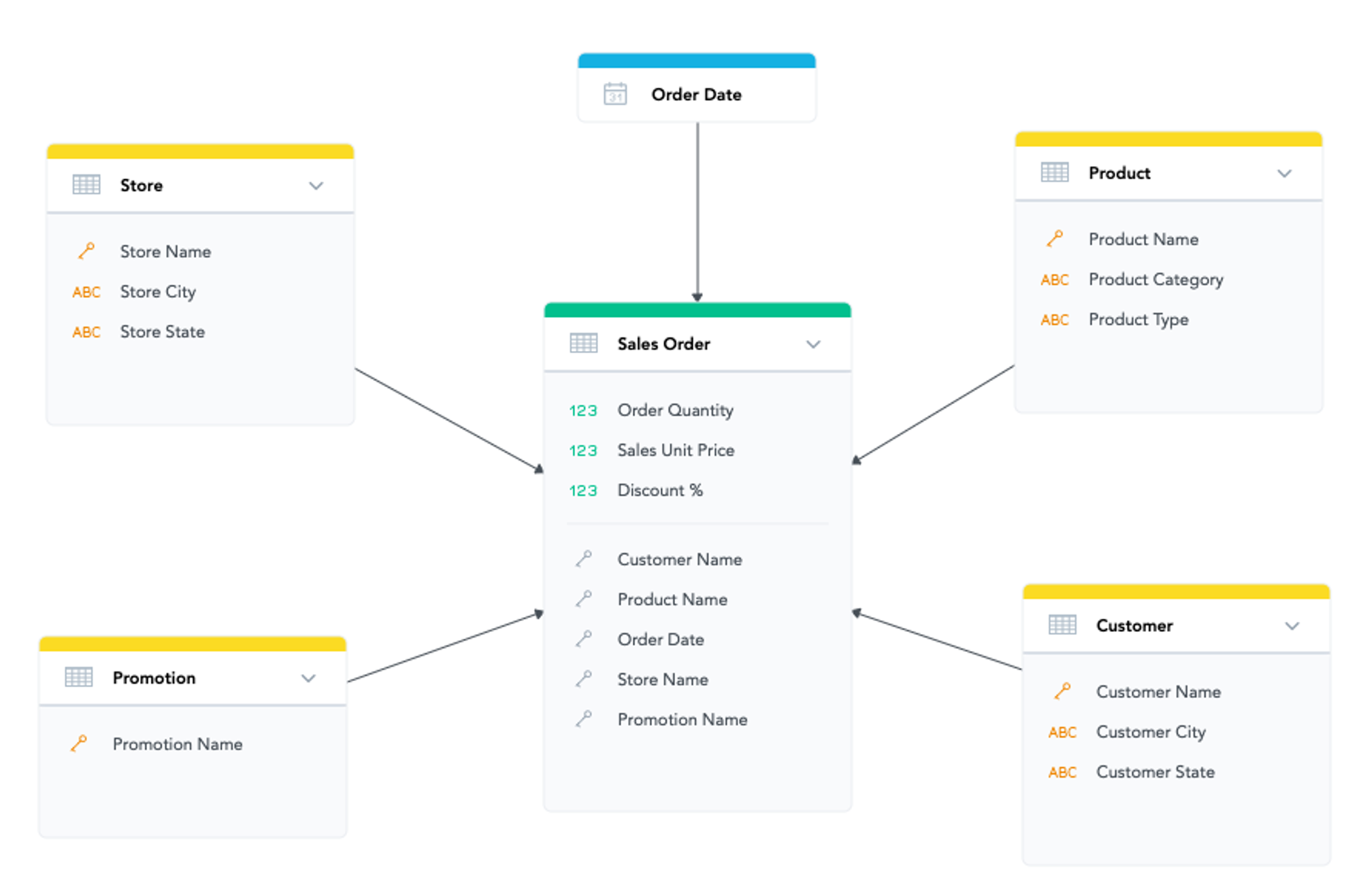
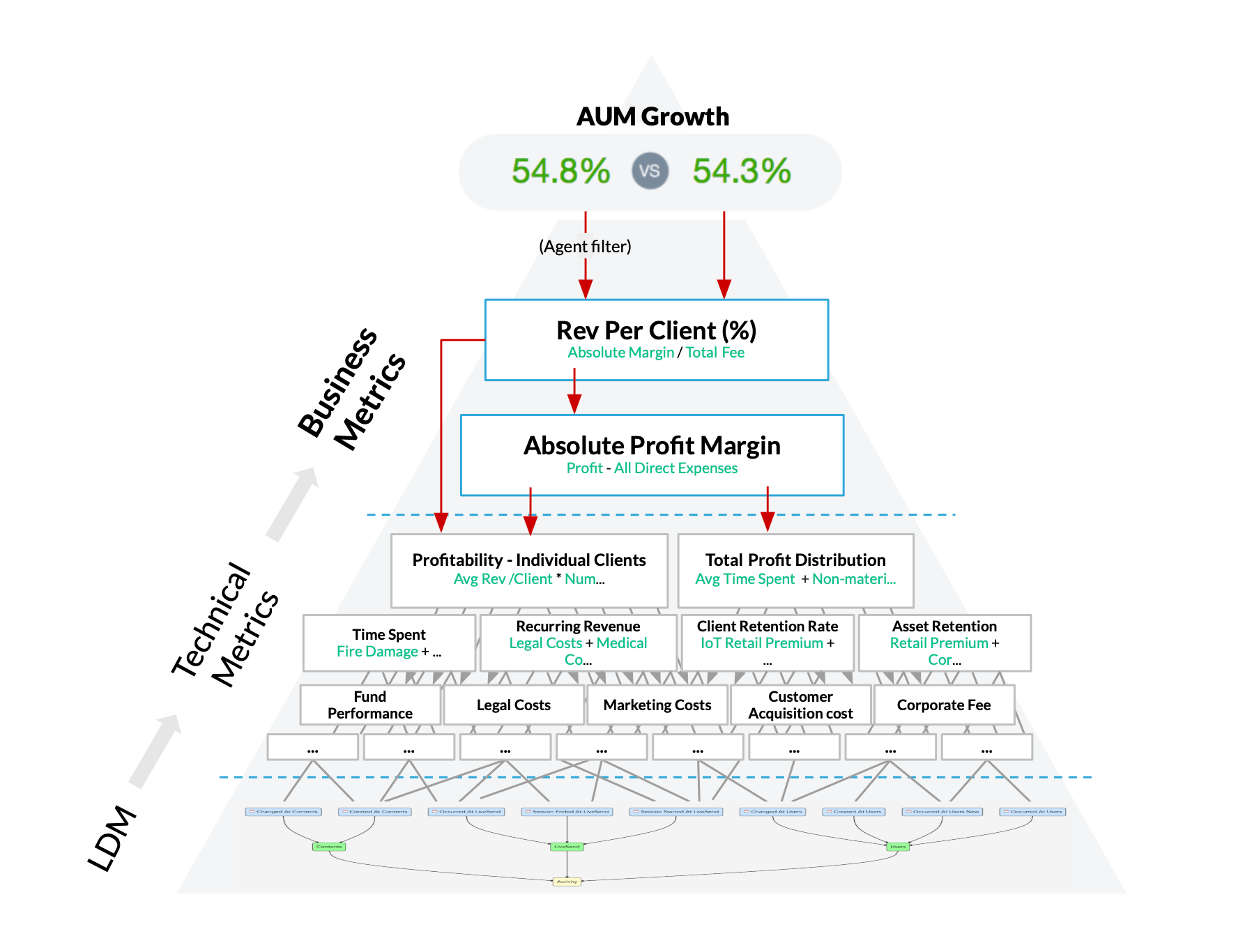
4. Eliminate Data Silos and the Need to Move Data
The Situation
With data being collected from multiple sources and moved between departments and applications, the prevalence of data silos and stale data is a common problem for companies rolling out analytics.
GoodData Solution
Your Snowflake data warehouse solves part of the equation by providing one location for storing all of your data from scattered data sources. The other half of the equation? GoodData Cloud to directly query your Snowflake data in real time for always up-to-date data analytics — without the need to move data while also eliminating data silos.
5. Avoid Metrics Inconsistencies
The Situation
As described above, with an analytics solution directly querying your Snowflake data in real time, end users always have access to the freshest data. At the same time, you avoid the need to move data. However, a successful analytics application will likely involve a diversity of users, analysts, developers, and data scientists who won’t be satisfied with just interactive data visualizations and dashboards.
They’ll want to use the analytics results in several other applications (e.g., BI tools, ML/AI notebooks, etc.) that form part of their workflow and combine these leveraged metrics with their queries. Instead of relying on outdated data exports, they’ll want to connect to the semantic layer and get real-time metrics, such as using their Python code with GoodData Python SDK.
Many companies approach this need by using multiple tools and platforms that sit on top of a shared database. However, ensuring analytics consistency across these various tools is difficult because each tool can use a different data model and query language as well as snapshots of data from different times. All of these differences can cause users to use ungoverned calculations in their tools. Unsurprisingly, this leads to data inconsistencies when four users report four different values of the same KPI.
GoodData Solution
Here is where headless BI is the solution. Headless BI enables end users to connect directly to the analytics engine embedded in your applications via standard APIs and protocols (e.g., JDBC or ODBC) to provide up-to-date, clearly defined data.
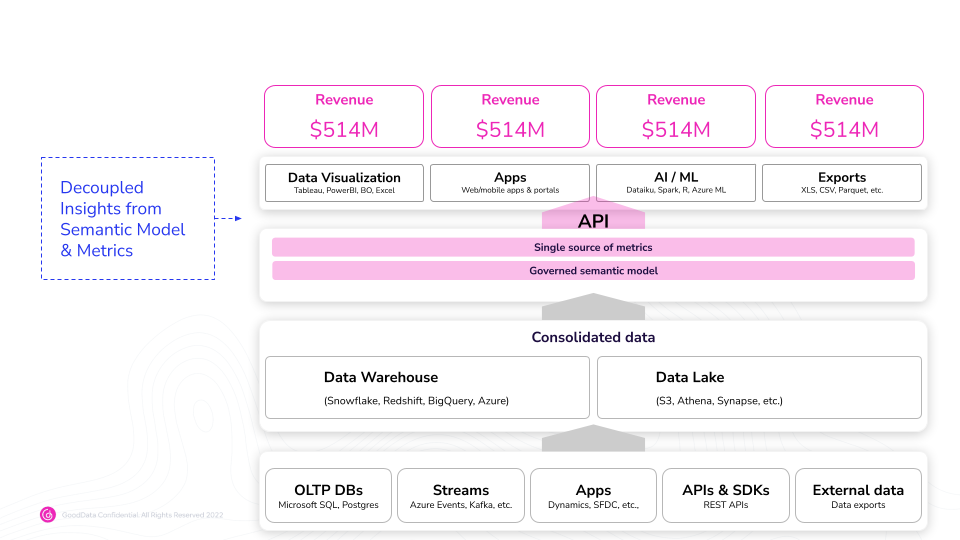
Try GoodData + Snowflake
Want to learn more about how to get the most out of your Snowflake data with GoodData? Read more about the benefits of our technical partnership or request a demo today and we’ll give you an in-depth guided tour.
Want to see what GoodData can do for you?
Get a guided tour and ask us about GoodData’s features, implementation, and pricing.
Request a demoWritten by Harry Dix |